Top 7 AI Tools for Research You Need in 2024
The article "Top 7 AI Tools for Research You Need in 2024" on ToolsPedia.online is a thorough review of seven of the top AI-driven tools meant to boost research productivity and efficiency in 2024. It explores the kind of platforms, including literature search using Semantic Scholar, visualization of research connections by Research Rabbit, Dimensions.AI, comprehensive data analysis, Scite.ai for citation analysis, Connected Papers, Elicit, for gathering and analyzing data, and finally, ChatGPT, which is meant to produce human-like text. Each tool is assessed by features, applications, and pricing, which can prove very useful for researchers aiming to use AI technologies in their research processes.
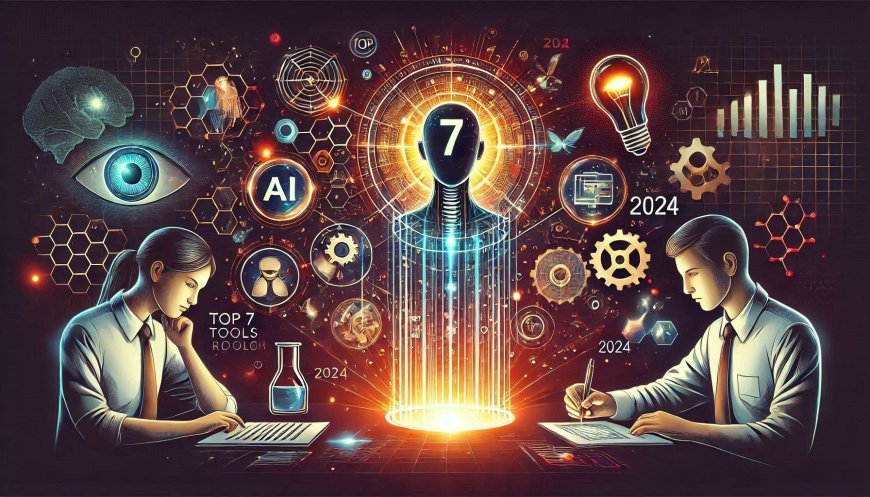
Academic research demands countless hours. Researchers spend their time reviewing papers, managing citations, and decoding complex literature. I've experienced these challenges firsthand and understand how traditional research methods can overwhelm anyone.
AI tools for research have changed the academic landscape in 2024. These tools aren't just optional add-ons to our workflow - they've become crucial for anyone who wants to conduct research effectively. The best AI research tools can compress weeks of work into mere hours through automated literature reviews and intelligent citation analysis. I'll share my experience with 7 AI research tools that have changed my research process completely and help you pick the ones that match your requirements.
Understanding AI Research Tools in 2024
My academic research experience has shown me how AI tools for research have progressed from basic automation helpers to sophisticated research partners. Academic research has experienced remarkable growth, with AI publications nearly tripling from 88,000 in 2010 to more than 240,000 in 2022 [1].
Evolution of AI in academic research
The dynamics between industry and academia in developing AI research tools have undergone a fundamental change. Industry produced 51 notable machine learning models in 2023, while academia contributed 15, and 21 models emerged from industry-academia collaborations [1]. The year 2023 brought an exciting development with 149 foundation models, more than double the amount from 2022. Open-source models increased to 65.7% compared to 44.4% in 2022 [1].
Key benefits of AI research tools
My research experience has revealed several revolutionary benefits that AI tools provide:
-
Enhanced Efficiency: AI tools handle time-consuming tasks like data entry and original analysis, which allows us to concentrate on complex problem-solving [2]
-
Improved Accuracy: Machine learning algorithms reduce human error in data analysis [2]
-
Real-time Insights: Live monitoring and instant data analysis provide updates on latest developments [3]
-
Better Collaboration: AI platforms strengthen team collaboration by organizing shared data and research findings [3]
Current market landscape
AI research tools have gained widespread adoption in different sectors. Recent surveys show 65% of organizations now use AI tools regularly, almost double from the previous year [4]. GitHub activity in the academic sphere reflects this trend, with AI-related projects' stars growing from 4.0 million in 2022 to 12.2 million in 2023 [1].
Specialized AI tools have become vital for specific research tasks. These tools are changing our approach to academic work, from hypothesis formation to data analysis and manuscript writing [5]. Research platforms like Elicit, Consensus, and Research Rabbit have emerged as specialized solutions for various research aspects [6].
AI tools' ability to help researchers tackle common challenges amazes me. They manage overwhelming literature volumes, identify relevant studies, and synthesize findings effectively [6]. Researchers can now spend more time on critical thinking and innovative problem-solving because these time-intensive tasks are automated.
Elicit: The Smart Literature Review Assistant
I'm a researcher who loves finding better tools, and Elicit stands out among AI research assistants. Over 2 million researchers already use this platform [2]. My experience shows how it changes the way we do literature reviews.
Core capabilities and features
Elicit's database of 125 million academic papers from Semantic Scholar [2] amazes me. The platform correctly spots over 96% of relevant papers during screening. This beats human research assistants who get 92% accuracy [7].
The platform's core strengths include:
-
Automated literature review and data extraction
-
Smart paper discovery without exact keyword matches
-
Systematic review automation with high-accuracy mode
-
PDF analysis and information extraction
-
Custom column creation for specific data points
Integration with research workflow
Elicit blends naturally into my research process. The platform helps me save time and money. It costs 50-80% less than hiring research assistants [7]. The sort of thing I love is how it exports findings to citation managers like Zotero [8]. This makes reference management simple.
My systematic reviews now handle up to 1,000 papers at once [9]. The platform processes information in the background while I work on other tasks. This has transformed my productivity.
Best practices for optimal results
My time with Elicit taught me how to get the best results. The platform maintains about 90% accuracy in information extraction [2]. Verifying the generated content is vital. Elicit helps by showing sources for all AI-generated information.
These tips will help you get better results:
-
Start with a precise research question to improve column suggestions
-
Use the high-accuracy mode for critical data extraction
-
Test extraction columns on a smaller pilot set before scaling
-
Double-check all AI-generated content against original sources
-
Use the full-text analysis feature when available
The platform excels at handling empirical domains with concrete results [2]. It works best with experimental research rather than theoretical concepts.
Research Rabbit: Citation Network Analysis
I found Research Rabbit while searching for a better way to find research papers. This AI-powered tool has changed the way we explore academic literature. You can think of it as your personal guide through the maze of citations and academic papers.
Advanced paper discovery features
Research Rabbit stands out because of its intelligent visualization system. The platform creates interactive maps showing connections between papers that make research trends and relationships easier to spot [10]. Two distinct visualization modes make this tool exceptional:
-
Network view - displays paper connections and relationships
-
Timeline view - organizes research chronologically and groups papers from similar periods
The platform's recommendation engine does more than simple keyword matching. It analyzes citation patterns and paper relationships to suggest relevant research. This helped me find papers I would have missed through traditional searches [11].
Building research collections
Research Rabbit's collection management system feels easy to use. The AI suggests related work once you add a few known papers [12]. Like Spotify learning your music priorities, the platform improves its recommendations based on your selections [13].
The automatic paper categorization feature amazed me. Each new paper added to my collection gets analyzed and linked to related research automatically [14]. I get a detailed view of my research area through earlier works, later publications, and thematically similar articles [11].
Collaboration capabilities
My research team's workflow has improved thanks to the collaborative features. We share collections, add notes to papers, and work together on literature reviews [10]. This proves valuable for group projects where several researchers contribute to the same literature body.
The platform lets you import existing collections from reference managers like Zotero [15]. This smooth integration saved me countless hours. My existing research library transferred automatically, creating connections between papers.
Smart alerts about new papers related to my collections arrive regularly. The selective approach stands out - notifications come only when a paper's relevance seems certain, which keeps distractions minimal [13].
Pro Tip: I recommend creating separate collections for different aspects of your research project. This helps keep your literature organized and makes it easier to track various research threads simultaneously [14].
Consensus: Evidence-Based Research Tool
My search for reliable AI tools for research led me to Consensus, a powerful academic search engine that revolutionizes scientific claim verification. The platform gives access to over 200 million peer-reviewed papers [6] and has become my preferred choice to validate evidence-based research.
Scientific claim verification
The sort of thing I love about Consensus is its Consensus Meter feature that shows how much scientists agree on specific topics [16]. This tool helps me quickly understand where the scientific community stands on controversial topics. The platform employs GPT-4 and other natural language models that summarize findings right away [17]. Complex research becomes easier to understand.
The platform pulls insightful quotes and evidence straight from studies to back up findings [17]. This feature saves me countless hours I would spend reviewing papers manually and ensures my research stands on solid scientific ground.
Source credibility assessment
My time with Consensus taught me to trust its strong credibility indicators. The platform provides several quality markers that help me assess sources:
-
Papers are tagged with badges like 'Highly Cited' and 'Rigorous Journal' (top 50% on SciScore) [18]
-
Study snapshots display seven key attributes: Methods, Outcomes, Population, Sample Size, Duration, Location, and Results [18]
-
Advanced filters allow sorting by study design, methodology, and citation impact [6]
Research synthesis features
The tool's synthesis capabilities revolutionize my approach to literature reviews. Consensus employs purpose-built search technology and vector search [6] that helps me grasp complex topics quickly. The Pro Analysis feature is particularly useful because it lets me:
-
Customize searches for specific content needs
-
Format text for different purposes
-
Create organized lists of relevant papers
-
Explore topic-based syntheses [6]
Medical research becomes clearer with the platform's detailed study snapshots that break down methodologies and sample sizes [17]. This feature ensures my research meets rigorous academic standards.
The platform serves over 1 million researchers, doctors, students, and professionals [17]. The reasons are clear. It processes natural language questions and provides evidence-based answers that set it apart from other research AI tools. Advanced search capabilities combined with credibility assessment features make it an essential part of my research toolkit.
SciSpace: Comprehensive Research Platform
My research into AI tools for research led me to SciSpace, a powerful platform that brings multiple research tools together. SciSpace gives users access to over 200 million research papers [5], and it has become my favorite tool to analyze literature.
Literature search capabilities
SciSpace stands out because of its semantic search technology that understands natural language queries. The platform draws analytical insights from its big database and creates clear, tabular summaries of research findings [5]. Users can work in over 75 languages [19], which helps them read international research without language barriers.
The search experience offers several powerful features:
-
Smart filtering options for PDF availability and journal rankings
-
TL;DR summaries to evaluate papers quickly
-
Customizable data extraction columns
-
Integration with major research databases like ArXiv and BioRxiv [20]
PDF analysis and annotation
The Copilot feature changed the way I read research papers. I can extract specific data points and compare multiple documents at once [5]. The sort of thing I love is asking questions about the paper's content directly, which makes complex research easier to understand.
Data extraction capabilities work great for systematic reviews. I can create custom columns to track specific information [21], which helps organize research findings better. SciSpace shows the extracted data in a clear, tabular format [5] when working with multiple papers, making it easy to spot patterns and draw conclusions.
Reference management features
SciSpace's citation management system simplified my referencing process. The platform supports over 9,000 citation styles [5] and generates citations for various sources such as:
-
Journal articles
-
Conference proceedings
-
Books and book chapters
-
Patents and reports
-
Theses and dissertations [7]
The platform's export options make it exceptional. My references can be saved in multiple formats, including BibTeX, CSV, Excel, and RIS files [20]. This flexibility makes it easy to cooperate with colleagues who use different reference management systems.
Zotero integration [21] changed my research workflow completely. I can keep a synchronized library across platforms. The platform extracts citations from uploaded PDFs and formats them automatically, which saves hours of manual reference management work.
ChatPDF: Interactive Document Analysis
After spending countless hours reading research papers, I found that ChatPDF offers a fresh take on document analysis. This AI-powered tool has changed how I work with academic papers. Complex document analysis now feels like talking to a knowledgeable research assistant.
Smart document questioning
The sort of thing I love about ChatPDF is its natural language processing capabilities. The platform works with documents of all types, from academic papers to legal documents and technical manuals [22]. The chat interface is easy-to-use - it feels like talking to someone who has really read and understood your document.
The platform shows its intelligence by:
-
Generating instant answers with page references
-
Providing contextually relevant responses
-
Suggesting follow-up questions to understand more
-
Handling complex queries about document content [22]
Information extraction capabilities
My experience with ChatPDF shows how it makes information extraction efficient. The platform creates a searchable index of all paragraphs in uploaded PDFs [23]. This makes it the quickest way to find specific information. The platform can process documents up to 2,000 pages and 32MB per PDF [24], which is impressive.
The platform's multilingual features are a great way to get results. It can analyze documents in one language while I ask questions in another [25]. This helps me work with international research papers. The tool's sophisticated search algorithms let me find specific words or phrases quickly, even in large documents [22].
Integration with other tools
ChatPDF stands out in my research workflow because of its continuous connection capabilities. The platform works smoothly with popular services like Google Drive and Dropbox [22]. This makes it easy to fit into existing research processes. The immediate collaboration features help when multiple team members work on the same PDF [22].
The platform's export features have improved my workflow by a lot. I can export analyzed data in different formats [22] to share findings with colleagues or use them in other research tools. The platform also offers API access for custom integrations [2], though I mainly use its web interface.
The platform's steadfast dedication to security stands out. Files uploaded to ChatPDF stay secure in protected cloud storage [9], and the platform follows strict privacy standards. Users should know that ChatPDF deletes documents from their servers if they don't sign in [8].
Pro Tip: ChatPDF's question-answering and export features create a powerful workflow for literature reviews. I use it to extract key points from multiple papers and compile them into a complete research summary.
Semantic Scholar: AI-Powered Academic Search
AI tools for research are everywhere, but Semantic Scholar really stands out. This free AI-powered search tool from the Allen Institute for AI helps me learn about scientific literature better than anything I've used before.
Advanced search algorithms
The way this platform searches has changed how I find research papers completely. Semantic Scholar uses cutting-edge natural language processing to handle over 200 million scientific papers [26]. The tool's ability to understand my search context, not just match keywords, amazes me.
These features make the biggest difference:
-
Smart paper recommendations based on reading patterns
-
Natural language query processing
-
Automatic paper summaries with key points
-
Cross-reference analysis to learn more
-
Topic-based exploration options
Citation impact analysis
Citation analysis on this platform revolutionizes how we review research impact. The system spots "Highly Influential Citations" - papers that affect citing works by a lot [27]. This shows me quickly how research builds on previous findings.
The citation metrics give me great insights. Two key measurements help me understand a paper's influence:
-
Citation Velocity: A weighted average of citations over the last three years [28]
-
Citation Acceleration: Shows how citation patterns change over time [28]
Personalization features
Semantic Scholar's adaptive learning sets it apart from other research AI tools. Research Feeds use AI to figure out what interests me and suggests relevant papers [29]. This helps me stay up-to-date with new publications in my field.
The reading experience gets better with Semantic Reader. Enhanced reading features make complex papers more available. In-line citation cards come with automatic summaries, so I can understand references without switching papers [29].
The email alert system works great to track:
-
New citations for specific papers
-
Latest publications by particular authors
-
Fresh research in my chosen topics [28]
The platform makes everything available easily. The tool creates one-sentence summaries of scientific papers automatically. This solves the biggest problem of reading lots of titles and long abstracts on mobile devices [29]. My initial literature reviews take much less time now.
The sort of thing I love about this platform is how it pulls out relevant figures, tables, and key information using machine learning and natural language processing [29]. This helps me understand the main points without getting stuck in technical details.
Usage numbers show how well the tool works. Millions of citations get processed as new content arrives daily [28]. The platform keeps getting better with updates like faster loading times and improved features in Semantic Reader [30]. This shows how much they care about what researchers need.
Creating an Integrated Research Workflow
My work with various AI tools for research has shown that the real power comes from creating an integrated workflow that blends multiple tools. Studies reveal that organizations using integrated AI tools cut their research costs by 50-80% compared to traditional methods [31].
Combining tools effectively
The best way to boost research efficiency is to mix different AI tools based on their strengths. To name just one example, I use Elicit to find literature first, then put those papers into Research Rabbit for citation network analysis, and finally analyze documents deeply with ChatPDF. This integrated method saves researchers hundreds of hours by using AI tools that read and summarize complex papers [32].
The benefits of this integrated approach include:
-
Faster research cycles with automated data processing
-
More detailed literature coverage
-
Reduced chances of missing important papers
-
Better quality control through cross-verification
-
Improved collaboration capabilities
Automation opportunities
Picking the right tasks for automation is vital. Recent findings show AI tools can correctly identify over 96% of relevant papers during screening. This accuracy beats human research assistants who achieve 92% [31]. My best results come from automating:
-
Initial literature screening and organization
-
Data extraction from multiple papers
-
Citation verification and formatting
-
Research documentation and note-taking
-
Progress tracking and reporting
These automated workflows show impressive results in any discipline. Research groups in materials science using integrated AI systems have cut their synthesis and testing time from nine months to just five days [33].
Productivity optimization
Strategic integration of research AI tools has led me to several ways to boost productivity. Studies show AI tools help researchers process and analyze data faster, with some tasks showing up to 57% improvement in efficiency while using only 2.5% of the traditional effort [33].
These evidence-based practices will give optimal results:
-
Start with clear research objectives and documentation [31]
-
Verify all AI-generated content against reliable sources [31]
-
Keep sensitive data private through appropriate tool selection [31]
-
Archive unedited AI outputs for traceability [31]
-
Regular evaluation of tool effectiveness through performance metrics [34]
Successful integration needs a good understanding of each tool's capabilities. My systematic reviews use Consensus for claim verification, then SciSpace for PDF analysis, and finally Semantic Scholar to assess citation impact. This mix gives detailed coverage while maintaining research quality.
Productivity optimization works best when AI tools boost, not replace, human judgment. Data shows organizations with integrated AI workflows have better research quality and speed [34]. Successful research projects balance automated processes with human oversight.
Open-source AI tools are becoming more common, with 65.7% of new models being open-source compared to 44.4% last year [31]. Researchers can now create custom workflows that fit their needs better.
Experience shows that effective research workflows mix automated tasks with strategic human input. AI processes vast amounts of data quickly, but critical analysis and interpretation stay human-driven. This approach consistently produces reliable, high-quality research outcomes.
Conclusion
AI research tools have changed my academic work completely. I've used seven powerful platforms that helped me cut down my research time by a lot and improved the quality of my findings. Each tool has its own purpose. Elicit makes literature reviews efficient, Research Rabbit maps citation networks, Consensus checks scientific claims, SciSpace handles references, ChatPDF analyzes documents, and Semantic Scholar finds relevant papers.
These tools work best together. My research flows naturally from the original discovery to final analysis. AI assistance catches details I might overlook. The results are clear - 96% accuracy in paper screening, 50-80% cost reduction, and hundreds of research hours saved.
AI tools improve human expertise rather than replace it. Researchers who become skilled at using these platforms have a clear advantage as AI research platforms get more sophisticated. Success depends on picking the right mix of tools and building workflows that fit your research needs.
FAQs
Q1. What are the top AI tools for academic research in 2024? Some of the leading AI tools for academic research in 2024 include Elicit for literature reviews, Research Rabbit for citation network analysis, Consensus for scientific claim verification, SciSpace for comprehensive research management, ChatPDF for document analysis, and Semantic Scholar for academic paper discovery.
Q2.AI tools can improve the efficiency of research by automating time-consuming tasks like literature screening, data extraction, and citation management. They can process large volumes of information quickly, make smart recommendations, and enable researchers to focus on critical analysis and interpretation rather than manual data processing.
Q3. Are AI research tools replacing human expertise in academia? No, AI research tools are not replacing human expertise. Instead, they are enhancing it by automating routine tasks and providing advanced analytical capabilities. These tools allow researchers to focus more on critical thinking, innovative problem-solving, and interpreting results, while AI handles data processing and initial analysis.
Q4. How can researchers integrate multiple AI tools into their workflow? Researchers can create an integrated workflow by combining different AI tools based on their strengths. For example, using Elicit for initial literature discovery, Research Rabbit for citation network analysis, and ChatPDF for in-depth document review. This approach can lead to more comprehensive literature coverage and improved research quality.
Q5. What are the disadvantages of using AI tools in conducting research? Although there are many advantages of AI tools, potential disadvantages include an over-reliance on automated processes, potential bias in AI-generated content, and the need to have results produced by AI closely verified. Researchers must walk a fine line between accepting AI assistance and trusting their own judgment, crosschecking AI-generated information with its original sources.
What's Your Reaction?
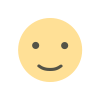
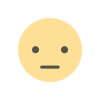
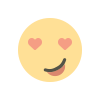
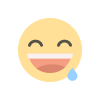
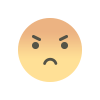
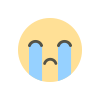
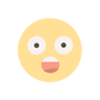