7 Free AI Tools for Literature Review You Need to Try
The article introduces seven free AI tools that can greatly enhance the literature review process for researchers and students. These tools apply artificial intelligence to streamline the collection, organization, and analysis of academic literature, making the review process more efficient and effective.
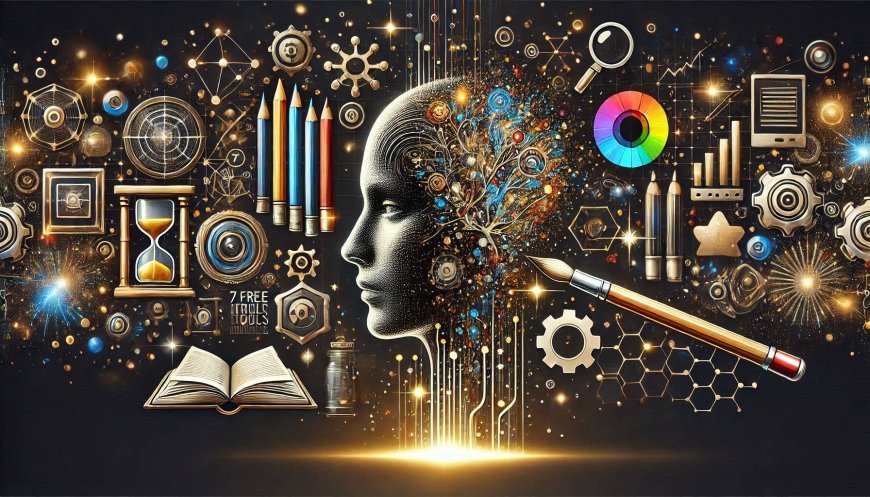
Literature reviews can take weeks or months of research time. I've spent countless hours going through papers and cross-referencing citations. The manual work of connecting different research threads has been time-consuming.
AI tools have changed everything about literature reviews, and that's what makes me excited to share this with you. These tools analyze hundreds of papers in minutes to extract key findings and spot connections we might miss on our own. I'll show you 7 free AI tools that revolutionized my research workflow. These tools will help you complete your literature review faster than before with features like automated paper discovery and intelligent summarization.
Understanding the Role of AI in Literature Reviews
My research experience has shown me how academic research has changed drastically. Knowledge production in research is accelerating faster than ever while becoming more fragmented and interdisciplinary [1]. Researchers now face bigger challenges to stay current with state-of-the-art research.
Rise of literature review processes
Traditional literature reviews often miss the mark because they lack a systematic approach, which leads to flawed research assumptions [1]. The growing volume of scientific literature makes it harder for researchers to keep pace [2]. AI has stepped in to reshape how we handle literature reviews.
How AI reshapes research workflows
AI has completely changed my approach to research. Science was one of the first fields where AI made its mark, and researchers quickly began to use these technologies in their work [3]. From my experience, AI tools now:
-
Analyze raw data at massive scales
-
Cross-reference papers across different journals worldwide
-
Extract higher-level themes and patterns
-
Identify research gaps and emerging trends
Benefits of AI-powered literature review
AI-powered literature review tools offer clear advantages to researchers. These tools process and analyze text much faster than humans and cut down the time needed for manual review [4]. They excel at searching multiple databases, repositories, and journals at once, which gives us better results than manual searches alone [4].
These AI tools automatically create summaries and combine key findings from literature. This helps researchers spot patterns across multiple studies quickly [4]. The tools also analyze large datasets to find gaps in current research and spot new trends. This knowledge helps us position our work better within the broader scholarly discussion [4].
AI tools bring another crucial benefit - they promote fair research practices. They remove human bias from search criteria and selection processes, which keeps literature reviews balanced and impartial [4]. This objectivity, paired with their ability to handle growing amounts of literature, makes them essential for maintaining quality research projects over time.
Streamlining Article Discovery with Research Rabbit
My search for quick research tools led me to find ResearchRabbit, a free citation-based literature mapping tool that makes finding papers feel as easy as discovering new music on Spotify [5].
Key features and capabilities
ResearchRabbit stands out with its visual approach to literature discovery. The network visualization helps me:
-
Map connections between papers and co-authorships
-
View research landscapes through timeline graphs
-
Track both earlier and later work related to my papers
-
Receive smart email alerts about new relevant papers
The tool's AI-powered recommendation system learns from papers added to my collections and improves its suggestions to match my research interests [5].
Setting up effective search parameters
Starting with the right setup makes a big difference. You should begin with 5-10 carefully selected seed papers that represent your core research area [6]. This focused starting point helps ResearchRabbit generate better targeted recommendations.
Your work can be organized into categories (broader subjects) and collections (specific topics) [7]. This hierarchical organization helps me manage multiple research threads at once.
Tips for organizing research collections
My experience has led to several strategies that maximize ResearchRabbit's potential. The collection system works like a digital research notebook. Each paper in your collection appears as a green node, while recommended papers show up in blue [8].
The sort of thing I love is knowing how to work together on collections. You can create shareable links and work with colleagues anywhere in the world [5]. Remote research collaboration becomes much more efficient this way.
Reading through abstracts before adding papers to your collection gives the best results. ResearchRabbit shows these automatically when you select the "abstracts" filter [8]. This ensures you build a high-quality research foundation instead of just gathering papers.
The uninterrupted integration with citation managers is one of my favorite features. Collections can be exported to tools like Zotero or Mendeley using BibTeX or RIS formats [6]. This integration saves countless hours of manual reference management.
Mastering Document Analysis with ChatPDF
PDF documents in academic papers can be quite dense. ChatPDF has become a great way to get more from my research toolkit. This AI-powered tool changed the way I work with research papers and get information from them.
Understanding PDF analysis capabilities
ChatPDF works like a smart research assistant who knows your document inside out. I found that it can process various document formats including PDFs, Word documents, and PowerPoint presentations [9]. The sort of thing I love is how it creates a semantic index of every paragraph. This lets me get quick and exact answers to my questions.
The tool shines with its easy-to-use interface that shows both chat and document side by side [9]. This setup helps me check information right away, which makes my research faster and more reliable.
Extracting key insights efficiently
My time with ChatPDF showed me several powerful features that make it great for literature review:
-
Instant document summaries and key point extraction
-
Natural language question-answering about document content
-
Direct citation links to source content
-
Multi-language support for international research
ChatPDF adds circular numbers to its responses that match specific pages in the document [10]. These numbers take me straight to the right section when clicked, which saves me lots of time checking sources.
Best practices for document interaction
My extensive use led me to develop some good strategies. Clear, specific questions work better than broad ones. The tool excels at finding exact information but needs focused prompts.
The tool has its limits. ChatPDF analyzes text well but can't understand figures or do statistical analysis [10]. I always double-check important information by hand, especially complex data or visual elements.
ChatPDF works best as an initial screening tool for academic work. It helps me quickly see if a paper fits my research by giving short summaries and answering specific questions about methods or findings [11]. This made my literature review much faster.
The tool's ability to look at multiple documents at once has worked great for me [9]. This helps me see connections between different papers and build a complete picture of my research topic.
Note that ChatPDF speeds up research a lot, but it should add to careful academic reading, not replace it. I use it as part of my bigger research plan, mixing AI features with traditional critical analysis.
Leveraging Semantic Scholar for Comprehensive Research
Semantic Scholar has become my go-to tool for literature reviews. This free, AI-powered platform changed my approach to finding and understanding scientific literature.
Advanced search techniques
Semantic Scholar stands out because of its sophisticated natural language processing capabilities. You don't need to struggle with complex Boolean operators. The platform's AI understands context and gives relevant results [12].
These tips will help you get better search results:
-
Use field-specific filters to narrow results
-
Apply publication date ranges for current research
-
Filter by publication type and author
-
Refine by journals and conferences
-
Focus on open-access content for full-text availability
Citation analysis features
The citation analysis tools revolutionized my research impact assessment. The Citation Velocity feature shows the weighted average of citations from the last three years [13]. This helps me spot trending papers in my field.
My paper analysis focuses on these key metrics:
-
Citation acceleration trends
-
Influential citation identification
-
Author influence scores
-
Citation context analysis
-
Citation graphs and networks
The sort of thing I love about Semantic Scholar is how it shows where and how papers cite references. This provides semantic context for each citation [14]. It helps me understand the purpose and application of citations beyond mere numbers.
Managing research recommendations
The recommendation system plays a crucial role in my research workflow. A free account lets me save papers and get individual-specific recommendations through research feeds [15]. Rating papers helps the AI learn my interests and improve its suggestions [1].
Email alerts help me track new developments in my field. These notifications keep me updated with recent research without constant manual checks [13]. The platform often leads me to relevant research I might have missed by identifying connections between papers.
The platform can process billions of citations [13], making it one of the most detailed research tools accessible to more people. It blends traditional citation metrics with modern analytics to show research impact more completely [14].
The TLDR (Too Long; Didn't Read) feature provides AI-generated summaries of publications [12]. This quick overview helps determine a paper's relevance before deeper reading.
Exploring Research Connections with Iris.ai
My research experience shows that Iris.ai takes a different path to literature review. The platform uses sophisticated AI technology to process over 70 million research paper abstracts from 3000 Open Access Databases [16].
Mind mapping research landscapes
The sort of thing i love about Iris.ai is its innovative fingerprinting technology. The system creates document fingerprints based on word-usage frequencies and combines various retrieval techniques, including vector database search, graph traversal, and keyword analysis [17]. This approach gives users a better grasp of research landscapes than traditional search methods.
The tool's visual interface has reshaped the way I look at research topics. I input a research problem or paper abstract, and Iris.ai creates a visual map that shows the main topics and matching documents [18]. This feature helps me save approximately 75% of my research time. It maintains above-human accuracy levels in specialized, interdisciplinary field analysis [19].
Discovering hidden research connections
Iris.ai's ability to uncover hidden research connections stands out as one of its best features. The system uses contextual 'fingerprint' matching of content with visualization tools [20]. It helps me find new views and map research areas, especially in new territories where exact terminology might be unclear.
These key features make my work easier:
-
Smart filters for rapid dataset reduction
-
Topic analysis for filtering
-
Concept selection for inclusion/exclusion
-
Context descriptions for similarity matching [20]
Optimizing search strategies
My experience has led me to develop better ways to use Iris.ai. The platform suggests building 15-18 maps over a couple of hours to get a full topic overview [21]. This method works better than spending equal time with traditional search engines.
Iris.ai's context-aware search capability makes it special. I can use my own description of what I need instead of simple keywords, and the system matches similar text across the dataset [21]. This helps a lot when exact key terms are hard to find.
The Analyze tool gets the best results for Topic and Word analysis and helps narrow down your dataset to relevant information [21]. The system processes both abstracts and full-text documents, which makes my research more complete and faster.
Accelerating Research with SciSpace
SciSpace has become my favorite platform to conduct detailed literature reviews. The platform gives me access to over 200 million research papers from various domains and disciplines [22]. Its powerful features have reshaped my research process.
Utilizing AI-powered search
SciSpace's intelligent search capabilities amaze me. The platform understands natural language queries through semantic and lexical search [23]. Matrix-style results make my work easier by extracting and organizing specific details about each paper—including findings, results, and methods [23].
These features make my work easier:
-
Live explanations for text, math, and tables [24]
-
Customizable search filters for publication type and year [22]
-
PDF availability tracking [25]
Managing research papers effectively
The document management system works like a charm. My articles and PDFs stay organized in a personal collection that's always available [24]. The platform stands out because I can upload my papers or entire folders to analyze [22].
Data extraction has changed everything about my workflow. SciSpace pulls relevant information from my PDFs and organizes it into customizable tables [26]. Dynamic and adjustable columns help me structure information based on my research needs [24].
Collaboration features
SciSpace's collaborative tools make team research projects efficient. Live co-authoring and commenting [27] have increased my team's efficiency. Discussion boards and communication channels make coordination with remote colleagues simple [27].
File sharing and project tracking work securely and effectively [27]. Our team's knowledge management system builds on previous work and makes research findings available quickly [27]. Large research projects benefit from this feature when multiple team members need to coordinate.
The Chrome extension fits perfectly into my daily research routine. It gives me instant insights and summaries about web content I browse [23]. This uninterrupted integration lets me analyze papers while browsing without switching tools.
The platform's Copilot feature helps me understand complex research papers. I highlight specific paragraphs to get detailed explanations [28]. Technical content becomes clearer and I can verify my understanding of specific concepts easily.
Maximizing Research Impact with Elicit
I've been learning about various AI tools lately. Elicit stands out as a real breakthrough for systematic literature reviews. The results are impressive - it can find over 96% of relevant papers when screening 5,000 papers. That's better than human research assistants who got 92% accuracy [29].
Research matrix creation
Elicit's Notebooks feature has become my go-to tool for organizing research. This platform lets me create detailed research matrices that save a lot of time during paper collection and analysis. The sort of thing I love is how it helps me pull data from papers systematically while keeping high quality standards for meta-analysis [30].
My proven workflow for research matrices looks like this:
-
Run multiple paper searches to build a detailed collection
-
Screen papers using customizable criteria columns
-
Extract relevant data using high-accuracy mode
-
Organize findings into well-laid-out tables
-
Export data for further analysis
Data extraction capabilities
Elicit has revolutionized my approach to literature reviews. The platform can save research groups 50% in costs and cuts time in half by automating data extraction that teams used to do by hand [3]. These features make it special:
-
Extracts data from both abstracts and full texts
-
Processes information from tables within papers
-
Creates custom columns for specific data points
-
Shows supporting quotes with context
-
Offers high-accuracy extraction mode for vital data
Custom analysis features
Elicit's custom analysis features have become essential to my research process. The platform searches through 125 million academic papers from the Semantic Scholar corpus [31]. This makes it great for empirical domains that use experiments and concrete results.
Custom columns in research databases add real value to my work. They let me track specific methods, rate evidence quality, and sort findings based on my research needs [32]. The platform works smoothly with citation managers like Zotero, which simplifies my process [29].
Elicit excels at handling empirical research, especially in biomedicine and machine learning [31]. The system makes it easy to check original sources and locate specific details within papers [31].
This tool shines with complex systematic reviews. Traditional reviews need 5 researchers and take 1.3 years to publish [33]. Elicit's automation cuts this time down while keeping accuracy high.
Meta-analyzes benefit greatly from the data extraction features. Adding new fields from 35 pre-defined columns helps me pull specific information across studies quickly. These columns cover study objectives, main findings, limitations, and theoretical frameworks [34]. This approach helps catch all critical information while maintaining research quality.
Best Practices for Using AI Literature Review Tools
My time learning about AI tools for literature review taught me that success depends on smart tool usage rather than just using them. My experience with many research projects led me to develop a method that gets the most benefit while steering clear of common mistakes.
Creating an effective workflow
The path to successful AI-powered literature reviews starts with a well-structured workflow. Research shows that AI tools help researchers process and analyze hundreds of papers in minutes [4]. This speed matters only when quality and accuracy remain intact.
Here's my tested workflow that gets results:
-
Start with clear research objectives and questions
-
Use AI tools to gather original literature broadly
-
Apply human judgment for relevance screening
-
Verify AI-generated insights against primary sources
-
Document your methodology to reproduce results
-
Regular backup and organization of findings
AI research tools work best to increase human effort, not replace it [4]. This method lets me utilize AI's speed while keeping the critical thinking needed for quality research.
Combining multiple tools effectively
Experience taught me that no single AI tool handles all parts of a literature review well. The best results come from finding seed papers with AI-based tools and then checking citation networks with citation-based tools [2].
My most successful approach combines these elements:
-
Tools like SciSpace or Consensus for original paper collection
-
Reference management with tools like Zotero or Paperpile
-
ChatGPT to filter and identify important papers
-
Obsidian for reading and annotation [2]
This method works well because it uses each tool's strengths while fixing individual weaknesses. To name just one example, AI-based tools might miss important papers, while keyword-based tools often give too many results [2]. Using both approaches gives better coverage.
Avoiding common pitfalls
Research experience showed me several mistakes to avoid with AI literature review tools. Results show that faster processing doesn't always mean better outcomes - people need to stay involved by looking at all AI-generated output and checking its value [4].
Quality improves when you check AI tool results against traditional library databases and Google Scholar [4]. This ensures complete coverage while keeping academic standards high.
Data privacy remains a significant concern when using AI tools. Many AI research tools let you opt out of data sharing and tracking history [35]. These privacy features protect your research data well.
Research reproducibility needs attention too. AI can analyze literature faster, but you need clear documentation of your methods. Studies show that AI algorithms often give slightly different results each run, which affects reproducibility [2]. That's why I keep detailed records of search strategies and selection criteria.
Custom GPT bots for specific research tasks improve efficiency by a lot [2]. These specialized tools process large volumes of papers while keeping analysis consistent.
AI tools work best as additions to traditional review methods [4]. They should help with specific needs in a combined workflow rather than being the only solution.
Research quality stays high when you verify all AI-generated insights with reliable sources. This means checking citations, ensuring data privacy, and looking for potential biases [35]. This extra verification step proves valuable for keeping research integrity intact.
Conclusion
AI tools have changed my approach to literature reviews. The process is now faster and more thorough than traditional methods. These seven tools - from Research Rabbit's accessible paper discovery to tools that bring out powerful data - have reduced my research time by half and helped me find connections I might have missed.
Each tool serves a specific purpose in my workflow. Research Rabbit finds related papers effectively. ChatPDF helps me learn complex documents quickly. Semantic Scholar delivers deep citation analysis. Iris.ai creates research maps. SciSpace enhances collaboration, while Elicit pulls out data with remarkable accuracy.
Success comes from using these tools strategically while you retain control. AI works best as a research assistant rather than replacing critical thinking. The combination of multiple tools and output verification gives me AI's speed and efficiency while ensuring academic research's quality and depth.
These tools show just the beginning of AI's research potential. Researchers who become skilled at using them will have a clear advantage. They'll produce complete, high-quality literature reviews much faster as these tools continue to improve.
FAQs
Q1. Are there free AI tools available for conducting literature reviews? Yes, there are several free AI tools for literature reviews. Some popular options include Semantic Scholar, Research Rabbit, ChatPDF, Iris.ai, SciSpace, and Elicit. These tools offer various features like paper discovery, citation analysis, and data extraction to streamline the literature review process.
Q2. How can AI tools improve the efficiency of literature reviews? AI tools can significantly enhance literature review efficiency by automating paper discovery, analyzing large volumes of text quickly, extracting key insights, and identifying research connections. They can process hundreds of papers in minutes, saving researchers substantial time while maintaining high accuracy levels.
Q3. What are some best practices for using AI literature review tools? Best practices include developing a structured workflow, combining multiple tools to leverage their strengths, verifying AI-generated insights against primary sources, documenting methodology for reproducibility, and maintaining human oversight. It's crucial to use AI as a complement to critical thinking rather than a replacement.
Q4. Can AI tools help in identifying research gaps and trends? Yes, AI tools are particularly effective at identifying research gaps and trends. They can analyze vast amounts of literature, map research landscapes, and uncover hidden connections between papers. This capability helps researchers position their work strategically within the broader scholarly conversation.
Q5. How do AI literature review tools handle data privacy and reproducibility? Many AI research tools offer flexible options to opt out of data sharing and tracking history to protect research data. However, it's important to note that AI algorithms may produce slightly different results each time they run, which can affect reproducibility. To address this, researchers should maintain detailed records of their search strategies and selection criteria.
What's Your Reaction?
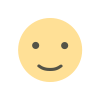
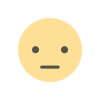
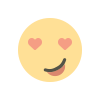
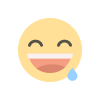
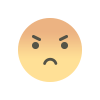
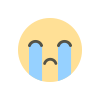
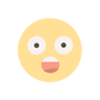