7 Essential AI Tools for Data Visualization in 2025
The article discusses seven essential AI tools that are going to transform data visualization for businesses and analysts in 2024. These tools tap into the power of artificial intelligence to simplify complex data sets, enhance visual storytelling, and improve decision-making processes.
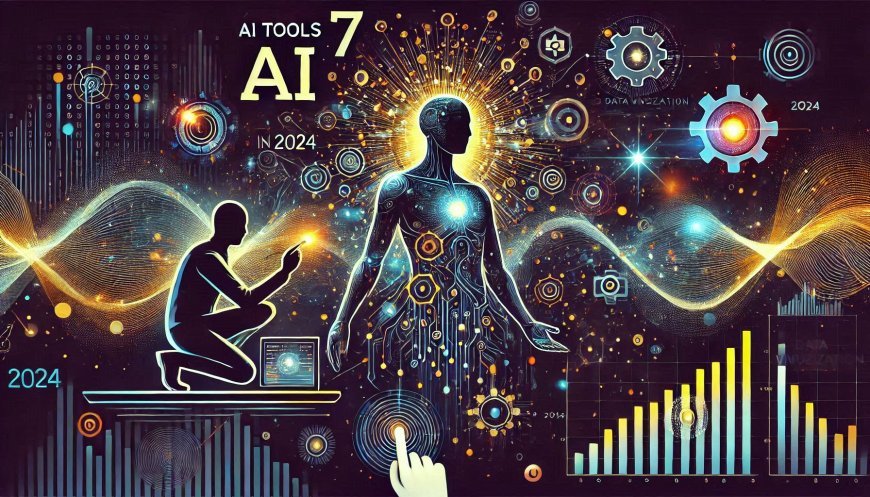
Complex data analysis once demanded hours of manual work and endless attempts to create meaningful visualizations. Everyone knows the feeling - staring at spreadsheets while searching for the best way to present information that truly matters.
AI tools for data visualization have now revolutionized the digital world. These powerful solutions turn raw data into compelling visual stories within minutes. AI visualization tools use automated chart suggestions and intelligent pattern recognition to transform how we understand and present data.
The top 7 data visualization AI tools of 2025 deserve your attention. You'll learn about their unique features and capabilities that help you pick the right solution for your needs. Data analysts, business leaders, and researchers will find practical ways to boost their data visualization workflow.
Understanding AI-Powered Data Visualization
The world has seen amazing changes in data handling and presentation. By 2025, global data generation will exceed 180 zettabytes, with 80% being unstructured [1]. This massive volume explains the need for powerful AI tools in data visualization.
Rise of data visualization tools
Data visualization started with simple statistical graphics in the 1800s. William Playfair's innovative charts and graphs are the foundations of modern data presentation [1]. AI visualization tools now process and analyze data live. These tools create dynamic, interactive displays that seemed impossible decades ago.
How AI transforms data storytelling
AI reshapes data storytelling in unprecedented ways. Manual analysis and interpretation dominated traditional visualization. Modern AI tools automate these processes while adding layers of intelligence. AI-powered platforms scan large datasets, identify patterns, and generate visual representations live with minimal human intervention [2].
AI has improved our visualization capabilities in these ways:
-
Automated Analysis: AI processes complex datasets and suggests optimal visualization formats
-
Interactive Exploration: Users can manipulate data live to break down different scenarios
-
Pattern Recognition: AI identifies trends that human analysis might miss
-
Natural Language Processing: Users can interact with visualizations through voice or text queries
Benefits of AI in visual analytics
Traditional vs AI-Powered Visualization:
Aspect |
Traditional |
AI-Powered |
---|---|---|
Speed |
Hours of manual work |
Minutes with automation |
Insight Discovery |
Limited by human capacity |
Improved pattern recognition |
Interactivity |
Static presentations |
Dynamic, live updates |
Personalization |
One-size-fits-all |
Tailored to user priorities |
AI improves predictive analytics in visualizations especially when you have complex data. These tools analyze past behavior and present likely outcomes in visual formats [2]. Users get a clearer picture of future trends. Natural language processing integration has made these tools available to non-technical professionals who can use insights without IT teams [2].
Experience shows that AI makes visualization smarter, not just faster. The technology learns from user priorities and behavior to personalize displays with relevant information [2]. This personalization has changed how people interact with data. Insights are more available and useful than ever before.
Evaluating ROI of AI Visualization Tools
Investing in AI tools for data visualization requires a careful look at the return on investment. Our experience shows that a complete ROI assessment must look at both tangible and intangible effects [3].
Cost-benefit analysis framework
A successful ROI assessment needs to look at both direct and indirect benefits. A full picture of cost-benefit analysis should look at:
-
Revenue effects and feature improvements
-
Security and privacy improvements
-
Process optimization metrics
-
Competitive advantages gained
Studies show that for every USD 1.00 invested in AI, companies realize an average return of USD 3.50 [4]. This impressive return comes from many factors, including streamlined processes and better customer experience.
Measuring productivity gains
Our teams have seen major productivity improvements through AI visualization tools. Customer support teams that use AI-powered visualization tools show a 13.8% increase in issue resolution rates [5]. The results are even better for new team members who saw up to 35% improvement in their performance [5].
Our tracked productivity metrics include:
-
Time saved on data analysis
-
Accuracy of insights generated
-
Speed of report generation
-
User adoption rates
Implementation cost considerations
AI visualization tool budgets need to account for several cost factors. Original deployment costs typically range from USD 37,000 to USD 100,000 for a mid-sized enterprise [6]. Our recurring costs usually fall between USD 7,000 to USD 20,000 and cover electricity, maintenance, and ongoing integration [6].
Implementation costs change based on several factors:
Cost Component |
Considerations |
---|---|
Hardware |
GPUs and processing power |
Software |
Licensing and customization |
Training |
Team upskilling |
Integration |
System compatibility |
The core team of data scientists who manage these systems earn an average salary of USD 94,000 [7]. Many businesses choose outsourced solutions to keep costs down while maintaining quality.
Small-scale implementations work well with SaaS platforms that offer AI visualization capabilities at lower price points. These solutions deliver great value and need less upfront investment in infrastructure and specialized talent.
GraphMaker Pro: Enterprise-Grade Visualization
GraphMaker Pro stands out in our analysis of AI visualization tools. This powerful platform combines advanced AI capabilities with resilient security features that make it perfect for organizations needing professional visualization solutions.
Key enterprise features
GraphMaker Pro's natural language processing technology impresses us. Users can interact with their data through conversational queries [8]. The platform excels at turning complex data sets into clear, interpretable visualizations and has over 30 different chart types [9].
We value these features:
-
AI-driven analytics that present data optimally
-
Advanced data manipulation capabilities
-
Live visualization updates
-
Security that ensures no data storage on servers [10]
Integration capabilities
GraphMaker Pro has complete integration capabilities. The platform supports multiple ways to import data:
-
CSV file uploads
-
Google Sheets integration
-
Direct database connections [8]
Teams find the platform works well because it makes shared work and live updates possible across departments. The system handles complex data sets while maintaining performance, which suits operations of all sizes.
Pricing and licensing options
GraphMaker Pro's pricing structure gives you flexible options that suit different organizational needs. The platform has several tiers:
Plan Type |
Price |
Key Features |
---|---|---|
Basic |
Single developer license [11] |
|
Pro |
USD 1,299/year |
5-developer team, full source code [11] |
Enterprise |
USD 2,499/year |
10-developer team, SaaS support [11] |
Enterprise+ |
Custom pricing |
11+ team members, advanced features [11] |
Enterprise+ plan comes with extra benefits:
-
SSO/SAML integration
-
Custom integration options
-
Dedicated support and training [10]
Data privacy and security make GraphMaker Pro different from others. The platform doesn't store data on its servers [10], which addresses a vital concern for enterprise users. Mobile compatibility lets teams create and edit visualizations anywhere [10].
DataRobot: Advanced Analytics Platform
DataRobot stands out as a powerful platform that blends automated machine learning with sophisticated visualization capabilities. The platform processes complex datasets and gives you analytical insights automatically. This makes it a vital tool in our data analysis toolkit.
Automated insight generation
DataRobot's automation capabilities cut down analysis time by a lot. The platform looks at our data, spots patterns, and creates extra features that boost model performance [12]. We find its knack for testing hundreds of models side by side most impressive. It uses techniques like cross-validation to check how well they perform.
The platform comes with these automated features:
-
Automated feature engineering for better predictive power
-
Cross-validation and model evaluation
-
Up-to-the-minute performance monitoring
-
Automated documentation generation
Predictive visualization features
DataRobot's Visual AI capabilities really shine. The platform offers tools that help us understand and check model performance:
-
Image embeddings for viewing projections in two dimensions
-
Activation maps that highlight important regions in visual data
-
Neural Network Visualizer for detailed model analysis [13]
The Feature Effects tool shows how feature changes affect model predictions. Feature Impact helps us spot which elements shape model decisions most [1]. Together, these tools give us deep insights into our data patterns.
Industry-specific solutions
DataRobot adapts well to different sectors. Here's how it tackles specific industry needs:
Industry |
Key Applications |
Benefits |
---|---|---|
Healthcare |
Clinical outcomes, staffing forecasting |
Better patient care, smoother operations [14] |
Manufacturing |
Equipment maintenance, demand forecasting |
Efficient operations, downtime prediction [15] |
Banking |
Fraud detection, risk management |
Better security, happier customers [16] |
The platform serves 70% of the largest U.S. banks [16], which proves its enterprise-level strength. Healthcare organizations use it to streamline claims management and payer operations [17]. Manufacturing companies rely on it for supply chain optimization and quality control [15].
DataRobot excels at handling multiple data types at once. You can work with time-aware data, images, geospatial coordinates, and natural language in one platform [18]. This flexibility makes it a great tool for complex analysis needs.
Automated feature engineering creates new predictive elements from datasets without manual work [19]. This automation pairs with detailed model interpretability tools. You can understand complex data relationships while keeping your analysis process transparent.
Tableau AI: Business Intelligence Leader
Tableau AI is reshaping our understanding of business intelligence. We found that Tableau's AI integration created a new class of analytics called business science that brings sophisticated data science capabilities to business experts [20].
Smart analytics features
Tableau's AI-powered analytics makes data exploration user-friendly. The Tableau Pulse feature creates individual-specific experiences based on each user's KPIs [21]. The platform stands out by presenting automated analytics in plain language and proactively suggests angles we might miss [22].
Key capabilities that add value:
-
Natural language processing for data queries
-
AI-assisted data transformation
-
Automated insight generation
-
Metric bootstrapping for easy tracking
Real-time dashboard capabilities
Tableau's up-to-the-minute data analysis reshapes our data interaction. The platform's Einstein Copilot, currently in beta, works as an AI assistant with two innovative features: AI-assisted data transformation and automated catalog descriptions [21]. This combination helps us prepare data and understand our sources better.
Feature |
Benefit |
---|---|
Metric Goals |
Easy progress tracking against measurements |
Pulse Analytics |
Proactive updates on data changes |
Smart Suggestions |
AI-driven visualization recommendations |
Natural Language Interface |
User-friendly data exploration |
Collaboration tools
Tableau's collaboration features are unique in the market. Team members can share data, ask follow-up questions, and forward visualizations to stakeholders who need information [23]. The platform's web editing and authoring capabilities let our teams work together efficiently by making changes in a central location [23].
Tableau makes data analysis available to everyone. The platform brings trusted generative AI to the entire ecosystem, making it available for everyone in our organization [22]. The system boosts productivity by reducing repetitive tasks through smart suggestions and in-product guidance [22].
Einstein Trust Layer integration gives us confidence in data security and privacy [22]. This trust layer lets us make use of AI without compromising security standards, a vital consideration for enterprise deployments.
Our tests show that Tableau Pulse changes how we interact with data by helping everyone put data at the center of decisions [22]. The system adds automated, plain-language explanations to dashboards in seconds, which improves our team's understanding of complex data patterns.
ChartAI: Simplified Data Storytelling
ChartAI stands out from all the AI tools we tested for data visualization. The tool makes complex data storytelling simple and straightforward. Teams without deep technical knowledge can create professional visualizations easily.
User-friendly interface
ChartAI's accessible interface impresses us with its simple design. The tool makes use of AI to create high-quality diagrams and charts with text prompts [24]. A clean, straightforward interface handles multiple project types, which makes it unique.
These key features grabbed our attention:
-
AI-driven chart creation with minimal manual input
-
Simple drag-and-drop functionality
-
Real-time preview capabilities
-
Smart suggestion system
Template library
ChartAI's template system is complete and adaptable. The platform helps create various visualization types:
Chart Type |
Use Case |
---|---|
Sequence Diagrams |
Process flows |
Gantt Charts |
Project timelines |
ER Diagrams |
Database modeling |
Mind Maps |
Concept visualization |
State Diagrams |
System workflows |
The AI engine automatically spots dependencies and optimizes timelines when we add or remove tasks [25]. This feature saves us hours of manual work and calculations.
Export options
ChartAI's export capabilities exceed our expectations. The platform gives us many options to share and download visualizations. We can fine-tune our charts before exporting [24] to match our exact needs.
The export functionality has:
-
Multiple format options: Download as JPEG, PNG, GIF, MP4, or PDF [26]
-
Direct sharing: Share via live links or social media platforms
-
Integration capabilities: Connect with various data sources
-
Brand consistency: Apply custom colors and styling across exports
Real-time collaboration makes the platform perfect for teams. Users get 100 credits with the free beta version [27] to test all features extensively. This makes it a great starting point for teams thinking about implementation.
ChartAI shines in the market because it focuses on data storytelling. The tool blends data, narrative, and visuals into compelling stories that drive positive change [28]. Our team can tap into the full potential of our data, making it available and actionable for stakeholders at every level.
The platform's synthetic dataset generation features are a great way to test and demonstrate concepts [27]. We can quickly prototype visualizations before using actual data, which makes our development process efficient.
Julius.ai: Interactive Visualization
Our recent exploration of AI visualization tools shows that Julius.ai brings a fresh point of view to data analysis with its innovative interactive visualization approach. The platform stands out because it knows how to simplify complex data integration while keeping its powerful analytical capabilities [29].
Dynamic chart creation
Julius.ai excels at generating visualizations through natural language prompting. The platform creates clean, professional-looking charts from simple text commands, which has substantially improved our workflow [30]. The system handles visualizations of all types, from simple line charts to complex statistical models.
The platform delivers impressive dynamic visualization features:
-
Time series forecasting with predictive modeling
-
KPI dashboard generation with up-to-the-minute updates
-
Anomaly detection and trend analysis
-
Interactive data exploration features
Custom visualization options
Julius.ai's wide range of visualization options impresses us. The platform supports many chart types [31]:
Visualization Type |
Application |
---|---|
Correlation Matrix |
Data relationship analysis |
PCA Analysis |
Dimensional reduction |
Heat Maps |
Density visualization |
Choropleth Maps |
Geographic data |
Dendrograms |
Hierarchical clustering |
Violin Plots |
Distribution analysis |
Julius.ai stands out because it knows how to improve visualizations based on user feedback. We can ask the system to make charts more visually appealing, and it responds with refined versions that maintain data integrity [32].
Data source connectivity
Julius.ai's data integration capabilities are remarkably detailed. The platform supports many file formats [33], including:
-
Spreadsheets (.xls, .xlsx, .csv)
-
Google Sheets integration
-
PDF documents
-
Text-based files (.docx, .txt)
-
Image files (.jpeg, .png)
The system handles multi-tab spreadsheets well. Users can reference individual tabs in their prompts for targeted analysis [33]. This feature helps teams work with complex datasets that span multiple sheets.
Natural language processing technology has changed how we interact with our data [34]. Team members can type their questions and receive instant visual responses instead of struggling with complex query languages. This capability helps people of all skill levels in our organization learn about meaningful patterns.
Julius.ai's approach to data privacy adds great value. The platform doesn't store data on its servers [35], which is vital for organizations that handle sensitive information. Quick export options to CSV or Excel formats help teams share data while maintaining security.
Julius.ai creates captivating animations that bring data to life [35], setting it apart from other visualization tools. These dynamic visualizations help teams communicate complex trends and patterns to stakeholders effectively during presentations.
Implementation Best Practices
AI tools for data visualization need careful planning and smart execution to work well. Our experience shows that proper implementation can determine if you create a thriving evidence-based culture or waste your investment.
Tool selection criteria
The right AI visualization tool starts with a full picture of your needs. Studies show organizations should look for AI solutions that excel in their priority areas. They should think about functionality, scalability, and how well it works with their current systems [2].
Here's our tested evaluation framework:
Criteria |
Key Considerations |
---|---|
Data Integration |
Compatibility with existing sources |
Security Features |
Privacy protocols and compliance |
Scalability |
Growth accommodation capacity |
User Interface |
Ease of use and accessibility |
Support Services |
Training and technical assistance |
Looking beyond obvious AI applications can bring huge benefits [2]. Teams should evaluate tools for both high-precision tasks that need detailed work and low-precision tasks that work with minimal context [2].
Team training considerations
Good training is vital for successful implementation. Research shows self-learning doesn't work well for industry-specific advanced AI skills [36]. These training elements are the foundations of success:
-
Structured Learning Programs: Custom curricula designed for specific roles and departments
-
Hands-on Practice: Ground projects and practical exercises
-
Continuous Support: Access to expert instructors and learning resources
-
Performance Tracking: Regular assessment of skill application
Corporate AI training programs have substantially boosted team efficiency. They eliminate rote work and make brainstorming sessions more productive [36]. Good training consistently delivers returns through improved productivity and state-of-the-art solutions.
Change management strategies
Successful AI adoption needs reliable change management. Studies show organizations don't deal very well with moving from siloed, low-efficiency environments to streamlined, merged systems [37].
We target three areas for effective change management:
-
Strategic Communication
-
Clear explanation of AI benefits and needs
-
Regular updates on implementation progress
-
Open channels for feedback and concerns
-
-
Stakeholder Engagement
-
The core team gets involved early
-
End-users provide regular feedback
-
Implementation plans include stakeholder insights
-
-
Adoption Support
-
Detailed documentation and resources
-
Dedicated support channels
-
Recognition of early adopters
-
People often resist change because they fear job loss or don't understand the technology [38]. Our transparent communication strategy shows how AI tools improve rather than replace human capabilities.
Small starts and success-based scaling work best. Pilot projects help test AI capabilities in controlled environments before wider adoption [39]. This approach lets us:
-
Verify tool effectiveness
-
Spot potential challenges early
-
Build confidence among stakeholders
-
Improve implementation strategies
Data quality matters most in visualization tools [40]. We put substantial effort into data cleaning and preprocessing to ensure accurate insights. This focus on data integrity helps maintain trust in AI-generated visualizations.
The sweet spot lies between automation and human insight. AI automates many visualization tasks, but human intuition and expertise remain vital for interpreting and contextualizing data [40]. This balanced approach maximizes both technological capabilities and human experience.
Conclusion
AI tools for data visualization have transformed our approach to analyzing and presenting complex data. Our exploration of platforms like GraphMaker Pro, DataRobot, and Tableau AI shows how these tools blend powerful analytics with easy-to-use interfaces to deliver quick insights.
AI visualization tools do more than just create charts. They reveal hidden patterns, automate routine tasks and enable teams to make analytical decisions quickly. Success comes from choosing the right tool, providing detailed team training, and managing change effectively.
Companies that adopt these AI visualization tools see clear advantages. They analyze data faster, learn more from their data and make better decisions. These tools prove valuable for businesses of all sizes through improved productivity and strong returns on investment.
AI visualization tools deliver the best results when aligned with clear goals and proper implementation plans. The path to success starts with a full picture of your needs. Teams should invest in training and grow their implementation based on early wins. This approach helps maximize benefits while reducing potential risks.
FAQs
Q1. What are the benefits of using AI-powered data visualization tools? AI-powered data visualization tools provide automated analysis, interactive exploration, enhanced pattern recognition, and natural language processing capabilities. It processes complex datasets quickly, identifies trends that may have otherwise been missed by humans, and allows users to interact with visualizations through voice or text queries.
Q2. How do AI visualization tools improve productivity in data analysis? AI visualization tools significantly enhance productivity by automating time-consuming tasks, providing real-time insights, and offering personalized data presentations. They can reduce analysis time from hours to minutes, improve issue resolution rates, and help even newer team members perform better.
Q3. What factors should be considered when evaluating the ROI of AI visualization tools? When evaluating ROI, consider direct and indirect benefits such as revenue impacts, security enhancements, process optimization, and competitive advantages gained. Also factor in implementation costs, including hardware, software, training, and integration expenses, as well as potential productivity gains and time savings.
Q4. How do enterprise-grade AI visualization tools differ from basic options? Enterprise-grade AI visualization tools like GraphMaker Pro offer advanced features such as natural language processing, real-time updates, and robust security measures. They typically provide more comprehensive integration capabilities, support for large-scale operations, and flexible pricing options for different organizational needs.
Q5. What are some best practices for implementing AI visualization tools in an organization? Key implementation best practices include carefully selecting tools based on specific organizational needs, investing in comprehensive team training programs, and developing effective change management strategies. It's also important to start with small pilot projects, focus on data quality, and balance automation with human expertise for optimal results.
What's Your Reaction?
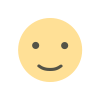
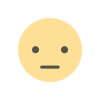
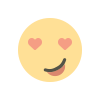
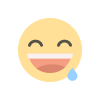
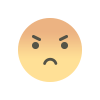
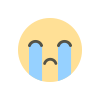
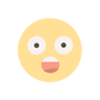